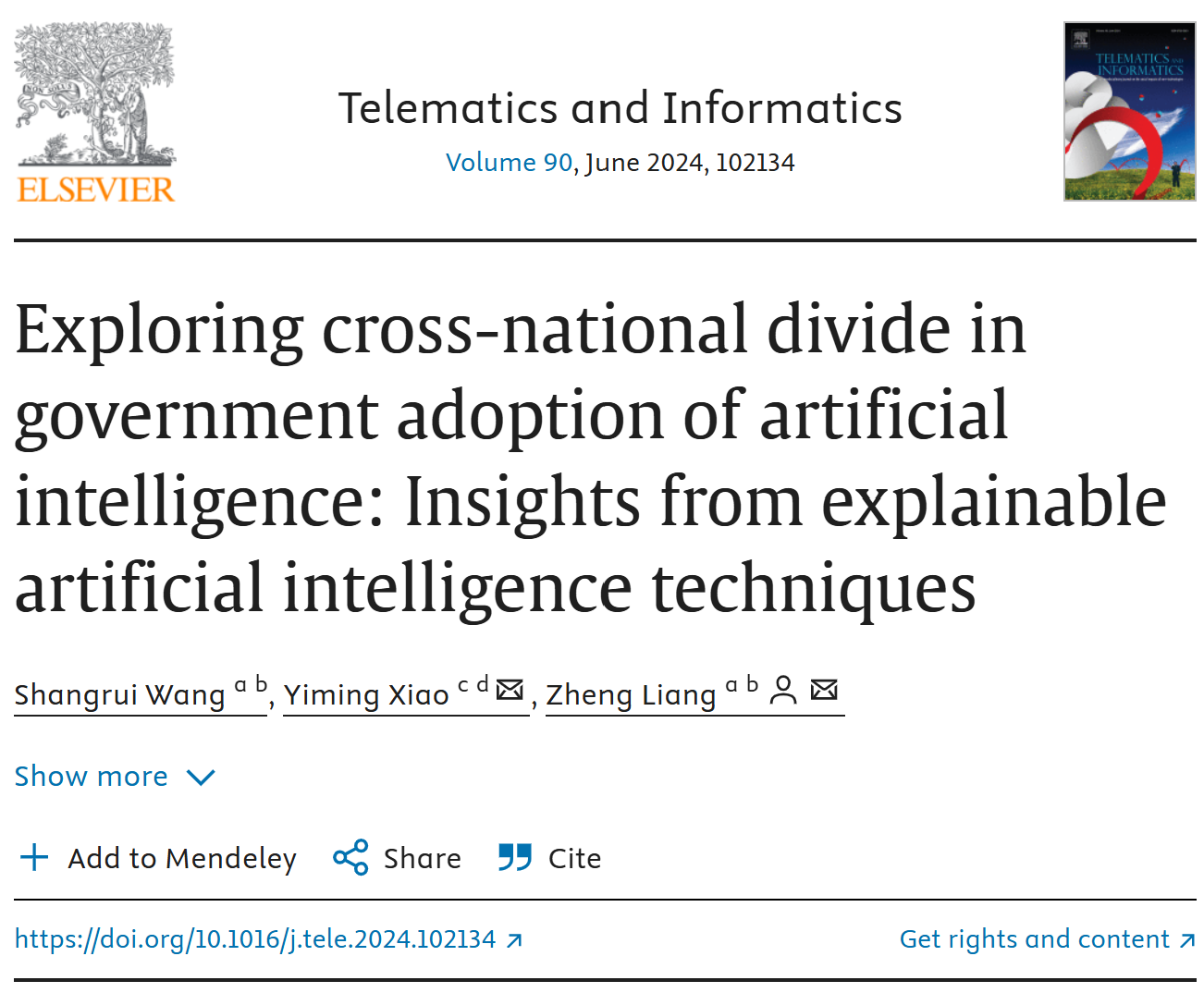
Highlights
•AI adoption is a continuum rather than a dichotomous.
•The underlying causes of global governmental AI adoption divide is explored.
•Explainable Artificial Intelligence (XAI) models are utilized.
•Technological infrastructure, institutional quality, finance resources, population structure, economic development and globalization are important.
Abstract
Despite the recognized potential of artificial intelligence (AI) to improve governance, a significant divide in AI adoption exists among governments globally. However, little is known about the underlying causes behind the divide, hindering effective strategies to bridge it. Drawing on the AI capability concept and the Technology-Organization-Environment (TOE) framework, this study employs Explainable Artificial Intelligence (XAI) models to analyze the multifaceted factors influencing AI adoption by governments worldwide. The results underscore the critical roles of internet security and internet usage within the technological dimension, regulatory quality, government effectiveness, government expenditure, rule of law, and corruption control within the organizational dimension, and globalization, median age and GDP per capita within the environmental dimension. Notably, our analysis explores the intricate effects of these variables on government AI adoption, identifying inflection points where their impacts undergo significant shifts in magnitude and direction. This nuanced exploration provides a comprehensive understanding of government AI adoption globally and illustrates targeted strategies for governments to bridge the AI adoption divide, making theoretical, methodological and practical implications.
Introduction
With an undulating trajectory from ‘preparadigmatic’ approach to ‘expert system’ to machine learning, AI is now becoming the most important disruptive technology leading the fourth industrialization (Kerr et al., 2020). AI can be broadly referred as an intelligent system with perception, process, decision-making, learning and adaption capabilities supported by software or hardware (Haenlein and Kaplan, 2019, Minkkinen et al., 2023). With the increasing recognition of AI’s immense potential in catalyzing economic development and advancing social progress, a growing number of countries have not only formulated comprehensive AI strategies but also actively adopted AI technology into public sectors. Therefore, AI deployment in public sectors transcends its role as a mere component of digitalization initiatives, emerging instead as a pivotal force propelling this transformation forward. It acts as a catalyst, enhancing both the pace and scope of digitalization through the integration of the most advanced technological innovations, which underscore a global governance paradigm shift towards leveraging AI to optimize governmental operations, enhance public services, and tackle complex societal challenges (Straub et al., 2023, Wirtz et al., 2019, Zuiderwijk et al., 2021). Several notable AI applications in public sectors encompass chatbot for government-citizen interaction (Maragno et al., 2022), algorithmic decision-making for automated administrative processes (Wang et al., 2023), intelligent security system for public safety (Laufs et al., 2020), environmental monitoring and disaster prediction system (Filho et al., 2022).
However, there is a significant disparity exists in the global adoption of AI in the public sector, with regard to the capacities, frameworks, expertise, resources, and infrastructure available. The Government AI Readiness Index reveals a huge gulf between the scores of 181 countries on a global scale. The United States of America has attained the highest position with a score of 85, whereas Afghanistan ranks at the lowest position with score of 13. The aforementioned gap is apparent not only in the instrumental perspective of efficiency, but also in the value perspective of responsibility.1 The current situation raises significant concerns, as the global gap in AI adoption has the potential to perpetuate and intensify global inequality, posing a threat the global AI governance (Sampath, 2021). While recent literature has extensively investigated the AI adoption in the public sector, they primarily focus on either the individual level (Ahn and Chen, 2022, Criado et al., 2022, Sun and Medaglia, 2019) or the organizational level (Mikalef et al., 2022, Neumann et al., 2022, van Noordt and Misuraca, 2022). Consequently, there is a limited body of knowledge regarding the factors underlying to the global AI adoption gap in the public sector, let alone suggestions for effective policies to bridge it. Against this backdrop, this study aims to address the following research question.
“What factors influence the AI adoption gap in the public sector on a global scale?”
The study applied the TOE framework to thoroughly seek theoretical explanation for AI adoption through technological, organizational and environmental dimensions (Tornatzky and Fleischer, 1990). While TOE framework is primarily employed in the context of organizational innovation adoption with a country, its applicability to global analysis has been substantiated (Adam et al., 2020), particularly in the field of digital government (Alhassan et al., 2021, Srivastava and Teo, 2007). Furthermore, the analysis employed Explainable Artificial Intelligence (XAI) models. XAI models are particularly pertinent in addressing the challenge of the ‘black box’ nature of AI algorithms, achieving high predictive accuracy and exceptional interpretability. These advanced models are instrumental in identifying the nonlinear impact of multidimensional factors on AI adoption to develop efficient strategies to bridge the gap in global AI adoption, while avoiding the need for predetermined assumptions regarding statistical distributions of residuals and the functional form of the equation (Stef et al., 2023).
The contributions of this study are threefold. First, this study is among the first to investigate the government AI adoption with global perspective, extending the existing literature in this field holding individual or organizational perspective. Second, this study draws on the concept of AI capability, considering AI adoption as a comprehensive outcome rather than a dichotomy. This study also tests the applicability of the TOE model in analyzing global AI issues. Third, this study employed the XAI model with ensembled decision tree algorithms and SHAP analysis, providing a novel methodology to investigate the importance of different determinants and their complex relationships underlying social phenomena according to predictive capacity.
The remainder of this study is structured as follows: After the introduction, Section 2 reviews the relevant literature and presents the theoretical framework. Section 3 describes the XAI models and dataset, and Section 4 presents the analytical results. Finally, Section 5 concludes with the main findings, theoretical implications, policy insights, and limitations.
Read more: https://www.sciencedirect.com/science/article/abs/pii/S0736585324000388