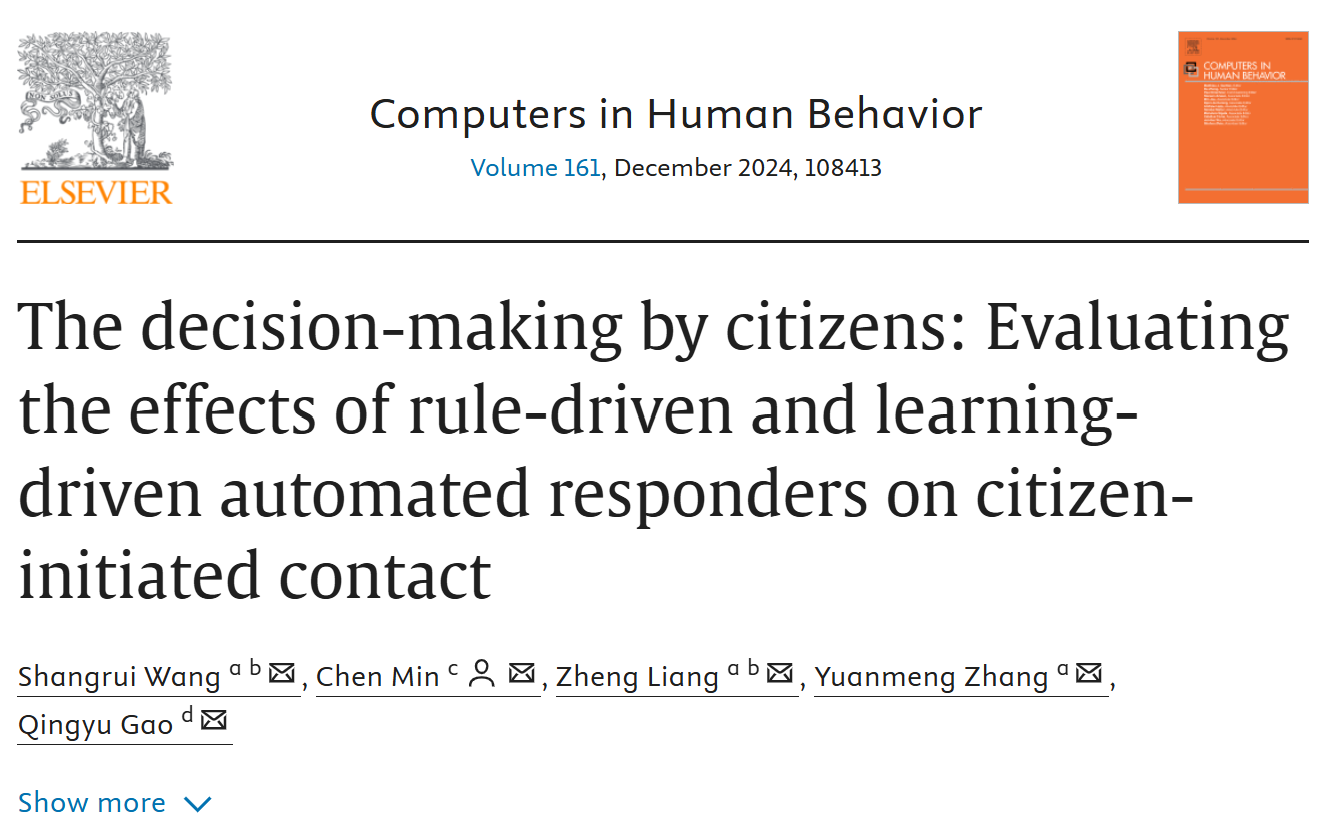
Highlights
•AI as automated respondents reduces citizen-initiated contact; learning-driven AI worsens the effect more than rule-driven AI.
•Respondent image, channel, purpose, and matter affect citizen contact but hardly mitigate AI's negative impact.
•Unlike the digital divide, young, highly educated individuals are least likely to contact AI respondents in government.
•The gap between AI adoption and social acceptance highlights the critical need for public participation in AI governance.
Abstract
While many studies have investigated the impact of artificial intelligence (AI) deployment in the public sector on government-citizen interactions, findings remain controversial due to the technical complexity and contextual diversity. This study distinguishes between rule-driven and learning-driven AI and explores their impact as automated respondents on citizen-initiated contact, an important scenario for public participation with initiative. Based on a conjoint experiment with 763 participations (4578 observations), this study suggests that AI deployments enormously reduce the likelihood of citizen-initiated contact compared to human response, with learning-driven AI having a higher negative effect than rule-driven AI. In addition, the causal effects of respondent image, contact channel, contact purpose, and matter attributes on citizen-initiated contact, as well as their moderating effects, are explored. These findings make theoretical implications and calls for public participation in the roaring AI deployment in the public sector.
Introduction
In recent years, the application of artificial intelligence (AI) in the public sector has been rapidly expanding to cover, but not limited to, internal management, public service, and social interaction (Sousa et al., 2019; van Noordt & Misuraca, 2022; Wirtz et al., 2021; Zuiderwijk et al., 2021), which has dramatically changed the form and outcome of government-citizen interaction (Akkaya & Krcmar, 2019; Aoki, 2020; Kankanamge et al., 2021). However, AI is a disruptive technology filled with developmental benefits and ethical risks (Busuioc, 2020; Taeihagh, 2021; Zhang et al., 2021), making AI applications in the public sector a controversial topic with AI enthusiasm and AI aversion. Even though AI has the potential to enhance the consistency and efficiency of public services and satisfy citizens’ needs for connectivity across time and space, it has been criticized for lacking warmth, trustworthiness and accountability, which harms the service experiences and expectations of citizens (Gaozhao et al., 2023; Ingrams et al., 2022). In this regard, the technical complexity of AI itself (Grimmelikhuijsen, 2022; Willems and Schmidthuber et al., 2022), as well as the diversity of application contexts (Gesk & Leyer, 2022; Willems and Schmid et al., 2022), are significant contributors to the discrepancy in the existing literature. Therefore, it is crucial to examine the impact of certain technological forms of AI on government-citizen interaction in a specific context.
Citizen-initiated contact with the government, where citizens contact the government with requests for services, advice, or complaints (Thomas & Melkers, 1999), is a starting point for government-citizen interaction and one of the main channels for public participation with initiative (Verba & Nie, 1972). The government has started using AI agents1 to generate citizen contact responses automatically. Global adoption of chatbots has been increasing over the last three waves of the UN E-Government Survey, with 69 countries now adopting chatbots, representing more than one-third of the global total.2 For instance, China's public sector has developed an AI boom during the last few years, as nearly all provincial-level and prefecture-level governments have implemented chatbots in their online portals (Wang, Zhang, & Zhao, 2022b). Simultaneously, local governments are gradually trialing the use of AI-powered robots offline and over the phone in addition to online services (Ju et al., 2023; Wang et al., 2021; Wang, Zhang, & Zhao, 2022b). These AI applications exhibit human-like intelligence through natural language processing, audio processing, and robotics technologies. However, they still rely on predefined explicit instructions from humans that are “formalized and reconstructed in a top-down approach as a series of ‘if-then’ statements” (Haenlein & Kaplan, 2019). In brief, rule-driven AI, also called as expert systems, replicates human behaviors by specifying top-down declarative logic (Haenlein & Kaplan, 2019; Janssen et al., 2022). Although it excels at responding to standard and commonly asked questions, it struggles to handle uncoded exceptions and to maintain and improve, leading to response failures (such as “Sorry, I cannot help you with that question”) (Ju et al., 2023). With the broad popularity of large language model3 (LLM) for human-AI communication, there is a growing consideration of deploying LLM in the public sector to automatically respond to citizen-initiated contact (Alexopoulos et al., 2023; Androutsopoulou et al., 2019; T. et al., 2022). In contrast to rule-driven AI that follows “if-then” instructions, LLM is a learning-driven AI that assimilates historical data, infers potential patterns, and generates pertinent information with minimal human intervention (Janssen et al., 2022). However, learning-driven AI has faced criticism for lacking comprehensibility, fairness, accountability, and legitimacy, ascribed to the complex and opaque computational procedure (Busuioc, 2020). In sum, significant differences have been observed between AI with predefined rules and AI with machine learning in administrative operations (Janssen et al., 2022; T. et al., 2022; Wang et al., 2023), as indicated in Table 1.4 Since citizens are crucial to upholding social values and dealing with ethical risks in AI governance (Wilson, 2022), this study categorizes AI as rule-driven and learning-driven, focusing on the impact of AI on citizen-initiated contact.
It should be highlighted that a variety of contextual elements influence how citizens express their preferences. According to the need-awareness model, citizen-initiated contact is dependent on citizen's needs for services and their awareness of the government's ability and willingness to respond (Jones et al., 1977; Thomas, 1982). Thus, supply on the government side and demand on the citizen side constitute the contextual elements of citizen-initiated contact. Following the “supply-demand” consideration, we propose that the image of the government respondent and channel for initiating contact on the government side, along with the purpose of initiating contact and the complexity and urgency of the matter on the citizen side, can exert substantial influence on citizen-initiated contact.
The causality is examined using the conjoint experiment. Compared to survey or field experiments, the conjoint experiment can examine multi-attribute causality feasibly that simulates actual decision-making situations and reduces social desirability bias (Hu et al., 2022). A final sample of 763 participants was recruited, with a total of 4578 observations to investigate causality.
This study makes several significant contributions to the field. Theoretically, it identifies the substantial impact of AI deployment on reducing the likelihood of citizen-initiated contact, with learning-driven AI having a more pronounced effect than rule-driven AI. It also elucidates how different contextual factors influence citizen-initiated contact and examines the moderating effects of these contextual factors and demographic variables. Methodologically, the study employs conjoint experiment design and Causal Forest techniques, demonstrating innovative and robust methods for analyzing complex interactions between government and citizens, especially when there are numerous substantive variables to explore. Practically, the findings provide actionable insights for AI development strategies in government, suggesting that AI should be used as a complementary tool rather than a replacement for human response, and highlighting the importance of public engagement in AI policy-making to ensure trust and legitimacy.
Read more: https://www.sciencedirect.com/science/article/abs/pii/S0747563224002814